Spatiotemporal modeling in transportation research
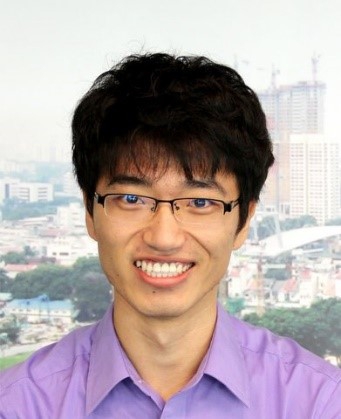
主讲人:Lijun Sun Assistant Professor
邀请人:马万经教授
时间:2020年9月25日(周五)10:00-11:00
地点:腾讯会议连接:https://meeting.tencent.com/s/KT7MCpvgQKJK
会议 ID:545 579 393
主讲人简介:
Dr. Lijun Sun is an Assistant Professor with the Department of Civil Engineering and Applied Mechanics. He obtained his PhD degree in Civil Engineering (Transportation) from National University of Singapore in 2015, and received his Bachelor degree in Civil Engineering from Tsinghua University in 2011. Prior to joining McGill, he was a Postdoctoral Associate at MIT Media Lab during 2015-2017 and a Senior Researcher at Singapore-ETH Center in 2015. His research focuses on intelligent transportation systems and urban computing, developing innovative methodologies, tools and applications to address the efficiency, resilience, and sustainability issues in urban transportation systems. He is now leading the smart transportation lab (http://smart.lab.mcgill.ca/) at McGill and the current research program of the lab centers on modeling large-scale, high-dimensional and spatiotemporal urban mobility and traffic data. Dr. Sun’s research has been supported by the Natural Sciences and Engineering Research Council (NSERC) of Canada, the Fonds de recherche du Québec – Nature et technologies (FRQNT), Canada Foundation for Innovation (CFI), and the Institut de Valorisation des Données (IVADO).
主讲内容简介:
Spatiotemporal mobility/traffic data, which registers timestamped travel activity/traffic state (e.g., flow and speed) observations from different locations (a network of sensors/zones), is an essential input to a wide range of intelligent transportation systems (ITS) and urban planning applications, such as travel demand prediction, trip planning, travel time estimation, route planning, ride sharing, transit service scheduling, signal control, and disruption management. These applications are critical components of smart transportation of the future, benefiting both travelers and transport agencies. The fundamental of modeling spatiotemporal mobility/traffic data is to characterize the higher-order correlations/dependencies within the data and perform efficient and reliable imputation/prediction for decision making. However, despite the abundance of modeling techniques, emerging issues are constantly challenging existing modeling frameworks, such as small-scale problem, linear system, and stationary, homogeneous and fully-observed data. As a result, in real-world online applications, these methods often suffer from limited scalability, failure in capturing multiresolution (short-range and long-range) dependencies, missing/non-stationary data problems, the limited number of sensors, and data heterogeneity. In this talk, I will introduce some emerging modeling frameworks on spatiotemporal data for both missing data imputation and traffic forecasting, and present some challenges for future research.
欢迎各位老师、同学参加同路人学术论坛!
交通运输工程学院研究生会
交通运输工程学院青年教师沙龙